Sorting problems with technology - is AI fantastic for plastic?
The potential opportunities and risks of artificial intelligence were explored at a British Plastics Federation seminar. Alex Brinded reports.
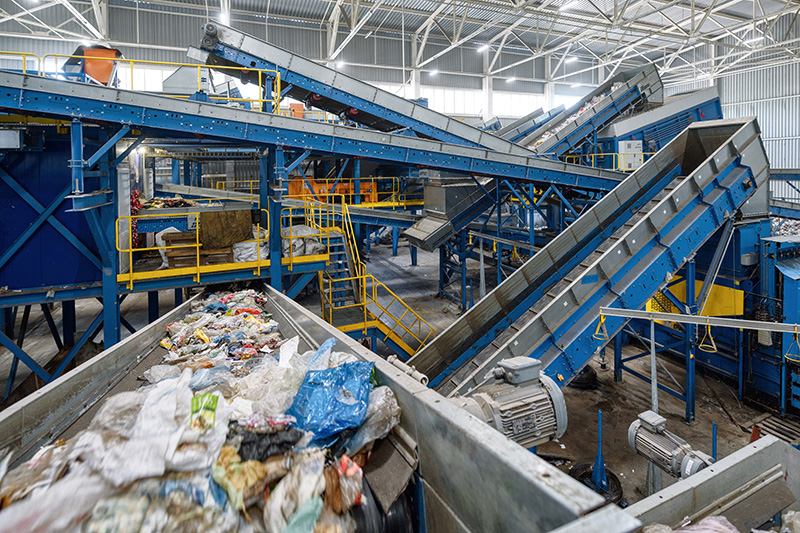
Ryan Gaffikin, an Associate at Dentons Global Advisors, presented a polished summary of the current EU and UK legislative landscape for artificial intelligence (AI) at a recent seminar hosted by the British Plastics Federation.
The EU’s proposed AI Act categorises different risk uses, he explained, from banned to regulated, lightly regulated and unregulated across all 29 member states. This applies to all EU suppliers, with the 'majority of the obligations falling on developers'.
The bill stipulates that, for high-risk use, developers must govern their own data, document key parts of it, maintain oversight and accuracy, and have robust cybersecurity. Exceptions include facial recognition AI for law enforcement.
But when to classify an AI system as high-risk has been a dilemma for policymakers, says Gaffikin. 'Negotiators are in the final stages of narrowing the scope now.'
With key disagreements remaining, the pre-Christmas deadline to pass the bill is now likely to be pushed forward to February or March 2024, with legislation coming into force two years later. If it is not passed before the June 2024 EU elections, the bill will probably get shelved, suggested Gaffikin.
He postulated to attendees that there is concern about EU overregulation among some companies, while others think frontier technologies need reining in early as it is impossible to predict how they will evolve.
The UK’s AI white paper released in March 2023, while not legally enforceable, is more sector focused, Gaffikin explained. This leverages sector expertise and creates flexibility, such as for financial services – which already sought regulator guidance.
The Chair of the Science, Innovation and Technology Committee, Greg Clark, has suggested a bill could pass before the UK’s 2024 elections.
The UK Government is thinking longer term, Gaffikin said, and is concerned about AI use for biological weapons or mass disinformation. However, Prime Minister Rishi Sunak believes it is too early to regulate, and it is necessary to generate solutions as risk of harm arises.
After the international summit held at Bletchley Park, UK, in early November 2023, the Frontier model taskforce was relaunched as the AI Safety Institute. Developers such as Open AI, Google and DeepMind pledged at the summit to provide early and priority access to models to understand and evaluate the risks.
This meeting affirmed international cooperation on research and allowed China and the US to sign the same communique.
In all cases, the primary responsibility for safety stands on the shoulders of the frontier AI developers.
Gaffikin noted that the UK Competition and Markets Authority is worried that the big players are already monopolising parts of the market, and smaller entities may struggle for investment and resources such as vast data stores. 'In the last year, we have seen that scale is what counts,' he reflected.
What’s in store for plastics?
For the plastics industry, AI can track inventory in real-time, provide quality control, design new materials, automate tasks, perform predictive maintenance, increase energy efficiency and reduce waste. Companies can simulate the factory floor in a digital twin to identify problems.
Chair of the event, Nigel Flowers, Managing Director of Sumitomo Demag, asked if UK owners of European equipment should be worried if legislation between the UK and EU diverges. Gaffikin clarified that, if, and when, the UK passes a bill, it can always be updated. He did note some Conservative Party pressure for a narrower bill, and that sectoral legislation leads to greater fragmentation.
Johannes Olbort, Project Manager at VDMA – an EU machinery and equipment manufacturers association – reflected that the hype around digital twins peaked in 2018, and now 'we are going from expectation to reality'.
He thinks hype around AI is just peaking, and that the basics still need to be understood. Artificial narrow intelligence can be language specific, such as Siri for iPhones, while artificial general intelligence is machine intelligence to solve a problem. Artificial superintelligence surpasses humans, he shared.
Olbort suggested the current hype is due to the exponential increase in data volume. He contrasted supervised learning – where a labelled dataset teaches AI – with unsupervised learning – when the dataset is unlabelled.
'We see a lot of opportunities, but it needs to be narrowed down to specific use cases and evaluated in a cost-benefit manner,” Olbort reflected. “If you don’t train the algorithms right, or have good data, bad input is bad output. There is no single good model.'
Asked if complete factory automation was possible, Olbort thought engineers would always need to supervise. 'People won’t accept what they don’t understand,' he concluded.
Quality control
Iain Smith, Managing Director of Fisher Smith in Northamptonshire, UK, with a background in plastics production, explained how the firm specialises in machine vision in manufacturing. It filters sub-par products from customers’ factory floors by spotting defects with cameras that monitor features, assembly and colour. Smith noted that an increase in using recycled plastic in products is causing more colour issues.
The cameras and learning algorithms also measure and help with sorting. The hardware and software can read date codes and manufacturing information, and in turn help trace defects to specific injection moulding machines, such as a worn drill bit.
'We are not generally dealing with full AI that makes…decisions…We are talking about a subset [that] gives us tools,' Smith explained.
He added that some products do not lend themselves to this method or have faults that are difficult to separate, such as different surfaces that have a uniform colour, or an error on a highly patterned product.
'What we have found is that over the last few years, the costs have been driven down and deep learning developments have really accelerated.' Data accumulation is now affordable, and a vast amount of computing power is embedded into industrial environments, he underscored.
However, some manufacturers are keen to keep their data off cloud services, Smith reflected, and technology has been developed to allow the deep learning model to be ‘trained’ locally with a processor or industrial device.
Price matters
Stephen Butler, CCO at London-based ChAI Predict, explained how the firm forecasts polymer prices with AI. Along with his two co-founders, Butler started the raw materials intelligence company after many years in the industry helping companies understand prices and plan budgets. The increasing volatility, especially since COVID-19, can have a destabilising impact on customers, he said.
ChAI’s web application currently focuses on metals, plastics and agricultural industries. They analyse different themes, such as supply, demand and alternative data – namely, satellites, weather and transport, for example.
The data is run through machine learning, and Chat GPT is often used to give the forecasts a narrative. As well as the most likely prices, ChAI provides best- and worst-case scenarios, mainly for manufacturing, distribution and training.
'Everyone has to explain [to their boss] why prices have gone up and down, so we are working very hard to incorporate market intelligence [analysing various datasets],' Butler said. This includes tracking tens of thousands of ships in real time as well as port activity.
The models work by building their learning data over time and so become increasingly accurate, explained Butler. They avoid inputting too much data to avoid creating 'weird relationships that can lead to black swan events'. But the 1,500-2,000 pieces of data embedded in the system are analysed daily – beyond what a market junior analyst can do.
ChAI recommends that customers do not to make seismic shifts based on this data, but factor it into their decision making. Responding to a question from the Chair regarding market instability, Butler said they don’t see crowd mentality events like financial markets as their customers have individual and unique use cases.
Waste analytics
Gaspard Dutihilleul, Head of Operations at Grey Parrot, London, UK, discusses how their technology uses AI for waste analytics. Started around five years ago, the company noticed a glut in product information pre-consumption contrasted with the deficit after consumption. In fact, there was just 0.1% of data coverage for total waste, due to most collection being done by hand. In a large waste ecosystem with multiple stages, they focused on sorting.
The team created an analyser to track and report on waste flows in recovery facilities. The 25kg piece of hardware can be fitted into different parts of the process, and contains cameras, lights and a processor.
This enables them to recover seven layers of detail about each product: material, mass, value, food-grade status and potential emissions – which they infer – and brand, the stock keeping unit and coordinates – relevant for sorting.
Dutihilleul explained how the model detects differences imperceptible to humans at speeds of 3m/s, from items that are damaged, overlapped and crumpled, and even black containers or when wrapping overlays an item.
They can also differentiate between food-grade and non-food grade objects for polyethylene terephthalate (PET) and high-density polyethylene (HDPE), with polypropylene (PP) in development. They now have 67 classes of plastic items that are ever expanding as the AI becomes more specific as it learns.
Christophe Parrot, Head of Sales at Recyleye, London, explained how his company provides quality control or sorting before the waste goes to a recycler. The company has optical sorting in 45 installations in eight countries, across HDPE lines, PET lines, paper, waste electrical and electronic equipment, metal, aluminium and residual waste.
They use labelled data to train the model with examples of products in different conditions, such as an HDPE bottle that has been crushed or shaped in many different ways. 'The more time passes, the more pictures it sees, the cleverer it becomes…It starts recognising commonalities in different products.'
Parrot explained that AI sorting and has different advantages and weaknesses compared to the conventional near-infrared method. For example, AI sorts black plastic and sleeved objects well, and differentiates non-food PET, like dishwasher bottles. Yet it struggles with transparent PVC bottles and small plastic flakes.
He feels AI is a complementary tool and could be trained to identify plastic films. When asked, he said that the software tends not to be impacted by different recycling schemes – such as those across the UK – but it is when plastic products are made differently, such as between the UK and France.
Cleaning up
The final use case of AI presented at the event was a software for mapping and tracking marine plastic.
Donal Hill, Co-founder of Plastic-i in Oxford, UK, described how the firm can detect plastic and marine debris to create a ‘floating debris index’ to automate reporting and identification at scale. Companies and policymakers would be able to review the impact of local policy changes alongside historical data.
The start-up uses data from polar orbiting satellites with a global coverage, as opposed to geostationary satellites, used mainly for telecommunications. Satellites use electromagnetic radiation to measure various properties of the Earth, and remote sensing can be passive by measuring reflected light, or active and by beaming down radio waves.
The sentinel 2 satellite sees in 13 different ‘colours’ – strips of wavelengths, covering the visible spectrum, and the near-infrared and short-wave infrared.
Hill explained how as anthropogenic waste has different properties, the relative reflectance informs the model about the contents. They take different bands to tune into indices such as the ‘normalised difference vegetation index’ and the ‘floating debris index’. 'In practice the AI algorithm figures out which bands,' he said.
Debris probability maps, where each pixel gets a probability scale, are a kind of segmentation model that also categorise spectral and spatial structures and detail object boundaries.
Plastic-i also forecasts the location of the debris after one or two days, using local wind speed and current information.
Hill said, 'The best way to fix this problem of ocean plastic is turn off the tap as far upstream as possible.'
Flowers concluded that a common challenge around AI is data integrity and tracking, 'I have been surprised by the real-time data that is available.'