Digging deeper - data infrastructure modernisation in minerals analysis
Jonathan Knapp, Geosciences Market Segment Manager at Bruker Nano Analytics, US, discusses automated mineralogy and the importance of data infrastructure modernisation in minerals analysis.
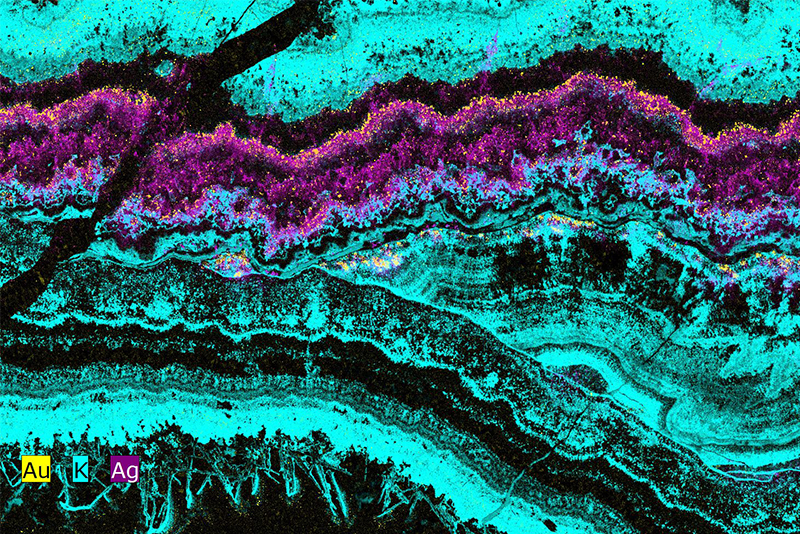
Where do you see an increased role for minerals analysis in the next few years?
The minerals industry is at the heart of the global economy, which is undergoing massive changes. A new generation has been challenged to decarbonise the economy and secure sources of critical minerals that can be produced sustainably and responsibly. The electrification of transportation and conversion of power generation will require a major increase in the supply and reliability of many metals and industrial minerals.
Automated mineralogy is unique in mineral analysis techniques in that it is a scalable assessment of mineral content and texture. Hundreds or thousands of samples can be characterised using a repeatable and quantifiable approach that captures not just what minerals are present but their relationship to each other.
The massive scalability of mineral texture analysis opens some truly revolutionary approaches to all aspects of the mining value cycle. While developing our AMICS software, we have focused on scalable workflows. For example, we take the approach that running a parallel batch reclassification is best, as it allows an unlimited number of files to be processed without needing to open them in memory. That is rather wonky, but it is just one example of how we can think about scale.
Operators can use automated mineralogy to make critical strategic decisions on data collected during the exploration and assessment phases. The combination of mineral, elemental and textural data allows for the assessment of not just the presence of ore, but also the characterisation of mineralisation style and refinement of the predictive ore body model.
However, the exciting opportunities are to plan for future operations. For example, we are starting to see projects to assess predictive modelling of mineral processing from the first radio controlled chips on a drill site. Mineral assemblages and textural associations can also be used to model acid generation from waste and tailings and better utilisation of those waste streams.
I see the biggest increase in the adoption of mineral analysis in assessing overburden and waste rock. Meeting the metals and critical mineral needs of the next great industrial revolution will require increasing the discovery and recovery rate for everything from copper to tantalum. The waste stream could be a low-cost and low-impact way of targeting minerals that would otherwise be sub-economic while enhancing the value of major projects.
Waste rock and overburden assessment can include the pre-feasibility study of overburden for possible commodities, or the re-assessment of historic waste and tailings piles for bypassed pay. Modelling the waste stream through space and time allows for better planning of future waste stream mineral availability. Utilisation or re-utilisation of this waste stream increases profitability.
What are the expected demands from clients?
Customers in the mining segment are always demanding – and I love it! Automated mineralogy is an important input into mission-critical processes. We need to make software with maximum up-time, a smooth workflow and impeccable reliability. The demands of the mineral industry are challenging, but they are always rewarding.
The demands around data and data access have changed in the last five years. Every major mining company is thinking about data in new ways, demanding that automated mineralogy seamlessly works with their data systems. They also demand that the raw data be delivered in its entirety. This is a revolution from the days of printing reams of paper with bar graphs. Companies now demand access to the spectra and to implement internal quality assurance/quality control procedures.
Data infrastructure modernisation was inevitable, as the data associated with mining has been ever-expanding. Geologists and engineers have collected a higher data density, and data sources have become richer and more valuable. Geochemists used elemental data in spreadsheets from acid digestion, X-ray fluorescence and fire assay. Now they may have hyperspectral remote sensing, overlapping geophysics surveys, core logs with high-resolution 3D images and X-ray fluorescence, as well as sub-micron automated mineralogy datasets. However, managing this data is challenging.
Is part of that challenge garnering meaningful insight from datasets that are getting bigger?
The problem with growing datasets is more than just technical it is also an issue of philosophy. The biggest challenge in data from automated mineralogy is making it accessible for advanced analytics. Machine learning and artificial intelligence could transform mining, but only if training datasets are available, accessible and properly formatted. Itʼs easy to throw up a cloud data storage location with some back-ups and say you have modernised data. I donʼt think this is enough. The data must be stored in structured, well-documented and curated form. That is why we have partnered with Hitachi to develop a solution for geochemistry analytics powered by Lumada.
Hitachiʼs solution for geochemistry analytics is a full-stack modern data solution that works with our AMICS data. First, the data undergoes an extract, transform and load process that transforms it into a structured and validated format.
Curated data is critical because it allows datasets to be compared, even if they are collected using different methods. Once loaded, the data can be categorised, grouped, or displayed within the platform. We have provided lots of data dashboards, a self-service data visualisation tool, and data can be accessed through an Application Programming Interface (API).
Accessibility is transformative for automated mineralogy – the original data (images and spectra) are available and can be transferred into a companyʼs central management system, and sub-sets can be accessed by an API or visualised directly in the system.
Data delivery and visualisation are important. One of the most common reframes I hear in the mining industry is that the exploration or drilling team is moving faster than the lab can work. I see it as a moral imperative to speed up the laboratoryʼs work to keep them relevant to the workers in the field. This means allowing critical data to be delivered to stakeholders as quickly as it is collected, validated and reduced.
What areas of R&D are you focusing on at the moment?
A new hybrid mapping mode can equally characterise fine and coarse-grained parts of the same sample.
We are focused not only on hardware and software but also on developing innovative methods. We are working on workflows that reduce the sample-to-results time and innovating new post-processing methods. For example, we have partnered with the Colorado School of Mines and the US National Science Foundation to develop methods for characterising historic tailings and waste rock with automated mineralogy.