Materials World is the flagship IOM3 members' magazine, specifically devoted to the engineering materials cycle, from mining and extraction, through processing and application, to recycling and recovery.
Editorially, it embraces the whole spectrum of materials and minerals – metals, plastics, polymers, rubber, composites, ceramics and glasses – with particular emphasis on advanced technologies, latest developments and new applications, giving prominence to the topics that are of fundamental importance to those in industry.
The magazine is widely accepted as the leading publication in its field, promoting the latest developments and new technologies.
Editorial content is delivered in a technical but accessible style, and keeps readers up to date with their industry, new trends, materials and processes.
The broad range of topics covered in each issue and the ongoing focus given to opportunities for individual personal development, make the magazine essential reading. Materials World carries display and recruitment advertising.
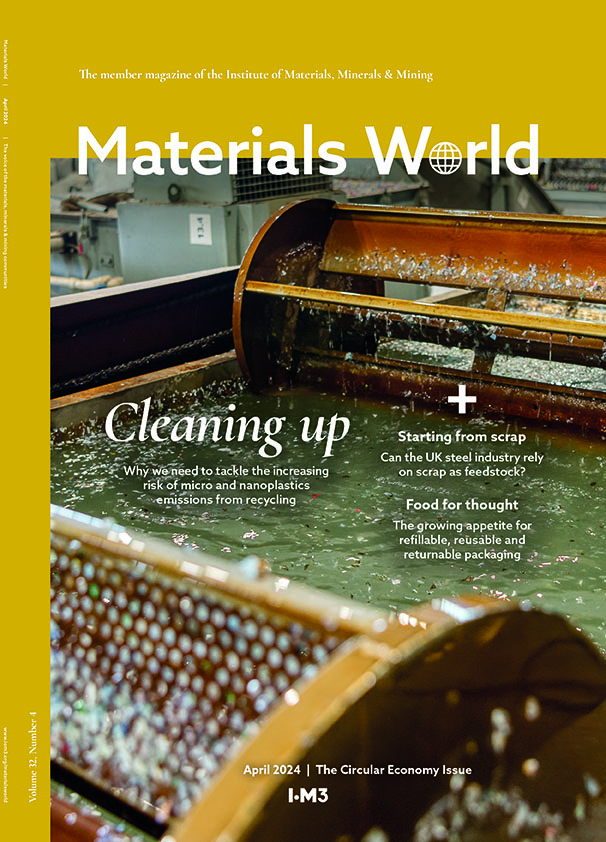
Read our latest issue
News, articles, opinions and profiles from the latest issue of Materials World. Please note that some articles are only available to logged in IOM3 members.