Digital Transformation: Chemistry and Advanced Materials Industry
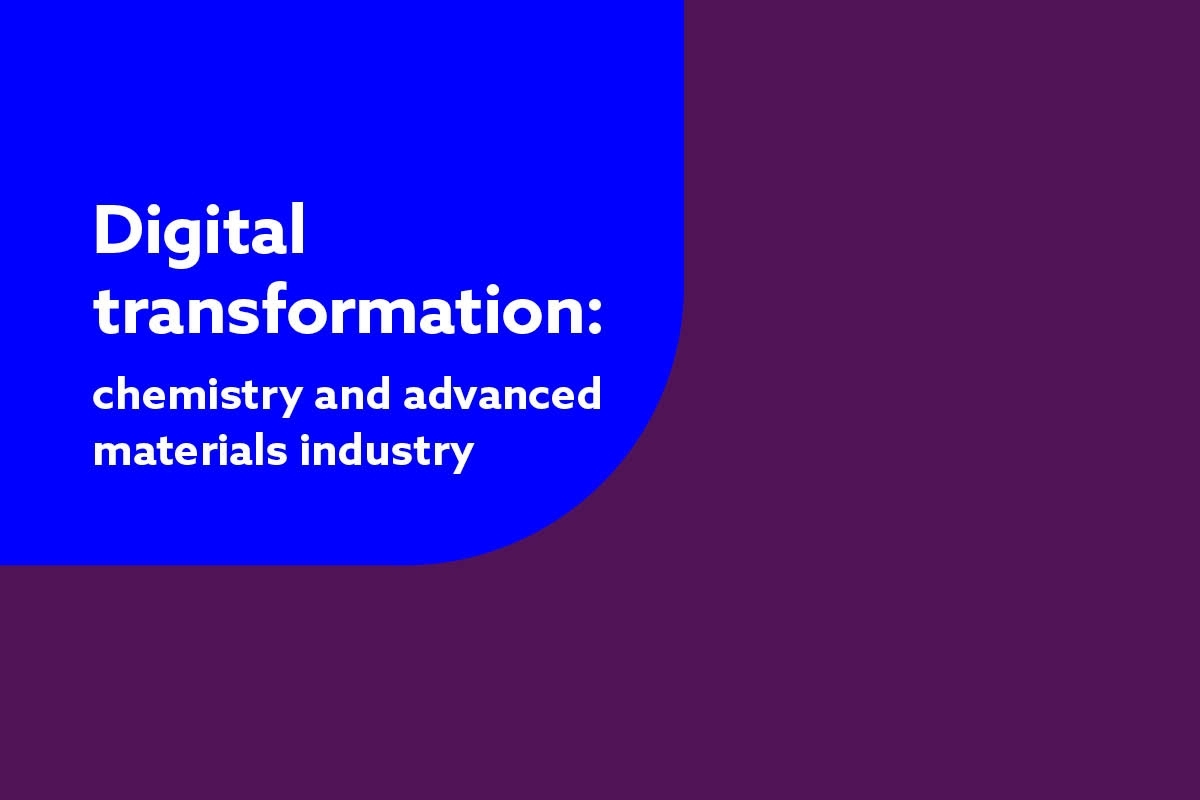
This webinar will focus on various theoretical ideas applied to machine learning (ML) such as density based clustering algorithms, game theory and simulations in conjunction with machine learning (ML) to guide materials design. An important part of the webinar will focus on how R&D industrial labs can transform from a traditional human-centric design to a digital-centric design.
You will learn:
-
Implementation of machine learning in companies.
-
Machine learning (ML) models for materials properties prediction.
- The use of simulations in conjunction with machine learning models of materials behavior to guide materials design.
Presentations on:
The elemental earth mover's distance between two inorganic compositions
Dr Matthew S Dyer, University of Liverpool
As materials scientists we are able to compare the composition of two materials and assess how similar they are likely to be in terms of their chemistry, structure and physical properties. It is, however, harder to define a quantitative and computable distance between two compositions which captures the chemical relationships between the two materials. Here we combine the earth mover's distance with the modified Pettifor scale to construct the Elemental Earth Mover's Distance (ElMD) which has these properties.
With the ElMD we are able to look in a database for the most chemically similar entries to a given composition, even if there a direct match is not present. The ElMD can also be used to compute the distances between thousands of compositions on a reasonable timescale, allowing the over 125,000 unique compositions in the Inorganic Crystal Structure Database (ICSD) to be represented on a single map. Focussing on binary compounds within the ICSD allows us to demonstrate how well the ElMD measures the chemical similarity between materials, showing that chemically similar binary compounds are grouped together with short distances between them and longer distances to chemically dissimilar compounds. This enables unsupervised learning of the chemical relationships between binary compounds using the density based clustering algorithm, DBSCAN.
Modelling dielectric constants with an explainable ML model
Dr. Morita Kazuki, Imperial College London
In the past decade, machine learning (ML) has emerged as a powerful tool in materials science, however, their predictions are often difficult to interpret, and their reliability was repeatedly questioned. In this study, we train a ML model on a dataset of dielectric constants and use game theory to derive the reasoning behind the prediction. We show that the ML model is capturing trends similar to conventional theoretical models, but with more generality.
Developing a digital-centric industrial R&D Lab
Michael C Heiber, Application Engineer, Materials Science Solutions Group, Enthought Inc.
Leading materials companies around the world are digitally transforming their businesses to keep pace with continuously evolving market forces, regulatory constraints, and emerging competitors. However, these initiatives have often been slow to penetrate the R&D laboratories that drive innovation and business growth due to the unique complexities and challenges inherent to scientific R&D. Nevertheless, industry leaders wish to leverage data-driven models to accelerate product development and lower costs by reducing expensive laboratory measurements. Based on our experiences, doing so requires transforming R&D labs from a traditional human-centric design to a digital-centric design. I’ll discuss a general strategy for how industrial R&D labs can begin transforming their laboratory processes and culture to be more digital-centric and tackle real problems to generate business value along the way.
Combining machine learning and simulations for materials design
Dr Bryce Meredig, CSO, Citrine informatics
Machine learning (ML) is often directly compared to traditional physics-based simulations in terms of accuracy in predicting materials properties. While such benchmarks can be valuable, casting ML and simulations as competing alternatives for performing the same tasks may cause us to miss opportunities to utilize both tools together. We will describe how these two computational methodologies are strongly complementary, and discuss examples of using simulations in conjunction with machine learning models of materials behaviour to guide materials design.
Data science to support material metrology
Recent years have seen the volume of data generation in materials science increase hugely. Simultaneously the variety of different sorts of data has grown.
The combination of volume and variety leads to new challenges and new opportunities in data science. The opportunities will lead to faster and more efficient analysis and development of new materials with desirable properties, innovative insight into complex phenomena such as degradation, and improved accuracy of prediction of material performance and lifetime.
The Data Science and Materials departments at NPL are working together to develop approaches, tools, and general guidance to enable researchers to make the best use of the data they gather. The talk will summarise the collaborative work based on the concept of a data lifecycle, including examples of successful projects and ideas for future work.