Smart sensor probes safer path to recycling nuclear fuel
A contactless smart sensor has been developed to better measure, in real-time, the acidity of highly radioactive solutions.
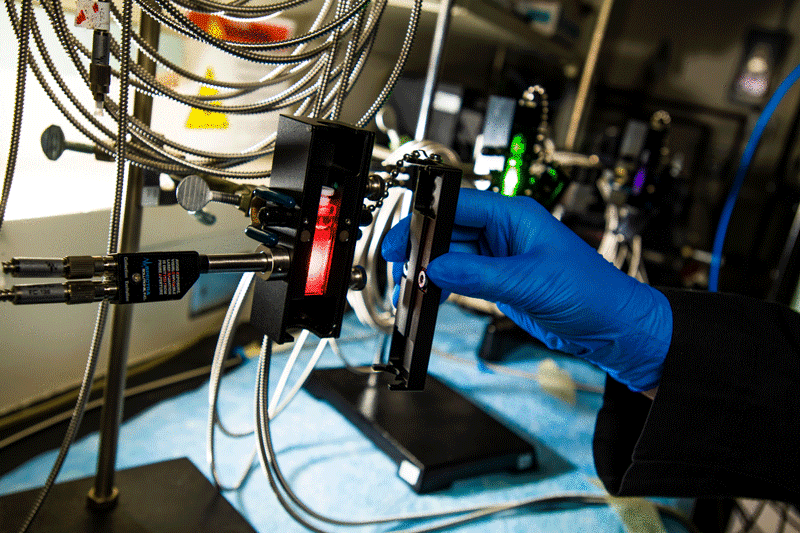
This could offer a more efficient and safer mechanism to process legacy waste and recycle spent nuclear fuel without opening, sampling, or otherwise handling the substance.
There is a growing interest to find a means to better determine the pH level and chemical structure of dissolved nuclear fuel after it has been fired in a reactor. The reason is that as the fuel is reprocessed to separate reusable fuel material from radioactive waste, variables like alkalinity and other chemical involvement can interfere with how well materials can be recovered and recycled.
In the first of two stages, researchers at the Pacific Northwest National Laboratory (PNNL), USA, have employed Raman spectroscopy to create a remote pH sensing technique for measuring how light interacts with chemical bonds, or optical monitoring of visible light spectra.
The advantage of Raman probes, say the scientists, is that they are commercially available, physically robust and can function for extended periods in harsh environments.
In the second stage, a machine learning technique, known as ʻchemometricsʼ, has been employed to create an algorithm to turn the spectral response into an acidity measurement.
The probes, explains Amanda Lines of PNNL’s research team, could be deployed for both contact (immersion into a solution or process line) or contactless (probe placed against a window into the solution or process line) applications.
Taking phosphoric acid as the demonstration species because it can ‘capture the complexity that can be anticipated in a real process’, the team has built the algorithms to allow for automated and accurate conversion of spectral data into information.
‘In a complex chemical system like the phosphoric acid solutions characterised here, accurately analysing data can be extremely difficult,’ describes Lines.
‘To help us overcome this, we use chemometric analysis. This involves collecting an optical library, or training set, of all the potential spectral fingerprints of the chemical species of interest. We then use this to train chemometric models that can calculate concentration or pH from observed spectral data.’
The team has incorporated math to compensate for acid/base pair ratios and activity coefficients of the phosphoric acid solution.
Under different levels of acidity, phosphate can take four chemical forms based on proton removal. PNNL’s technique quantifies each phosphate type and the total phosphate, at any pH.
‘Our probes output information in real-time, meaning product quantity and material accountancy can be immediately corrected or addressed as needed, again offering cost savings to the operation,’ suggests Lines.
Typically, in the nuclear industry, workers manually collect a sample to characterise chemical composition or measure pH. The problem is that nuclear fuel recycling or waste processing is a dynamic process, so this manual sampling can only offer limited or periodic insight.
‘This sample handling can pose safety hazards to workers and is slow, meaning it can take hours to months to measure and understand what is happening in the complex process,’ explains Lines.
According to PNNL, scaling up the findings for industrial applications is fairly straightforward. ‘The type of probe design used here can be integrated into a variety of process vessel or line sizes, and instruments can be optimised to monitor multiple locations with the process simultaneously,’ says Lines.
‘The larger challenge is preparing chemometric models to handle the increased turbidity of larger-scale process streams, [but] this is something that we have been developing and optimising through other project work.’